No models are perfect, but in drug discovery, the models are terrible. This is widely understood yet widely disregarded. Finding new drugs requires sifting through the practically infinite space of possible molecules to find the one that is very specifically going to nudge a human's chaotic biology in a very specific direction. Doing this faster and cheaper is core to human flourishing. Yet, innovation in how we perform this search is lacking and often misdirected.
Finding drugs would be hard enough if you could test directly on humans, but you don't get to test on your target system. So you use models which you hope approximate the relevant human target. But the closer the model system is to humans (e.g. primates) the cost in time, money, and overhead rises non-linearly. Which leads to relying on simple models that you hope tells you ~something~ and that the poor predictive power can be overcome with gobs of throughput.
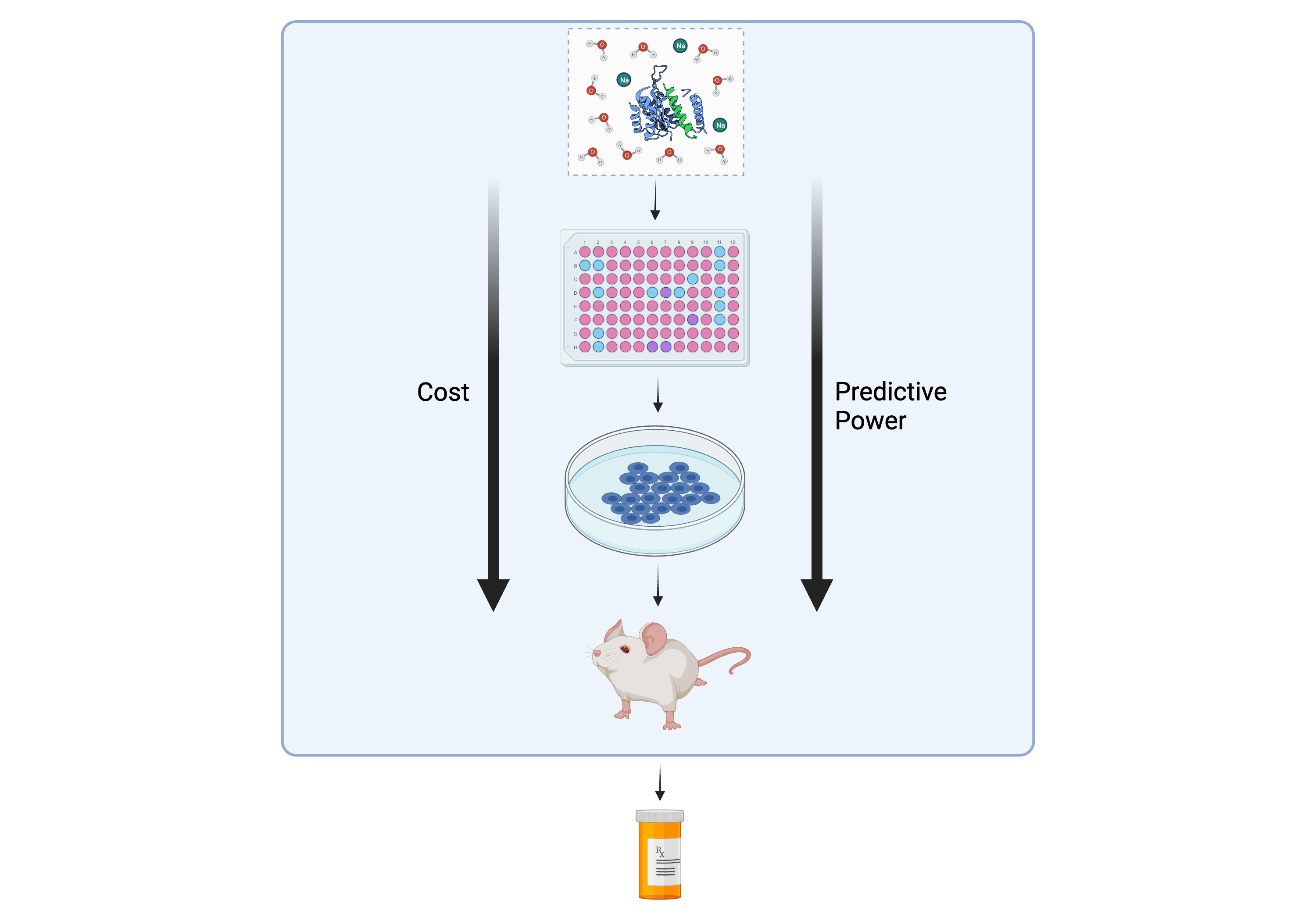
Biotech uses a magic potion of allegedly known biology, computer simulation, in vitro (think test tubes), in vivo (think animals), and optimism to find potential chemicals to eventually test in humans. This mostly fails, but it's not hard to diagnose why. Throughput of simple systems has exploded in the last couple of decades yet the trend-line for cost and success rate in discovering new drugs is solidly in the "yikes" category. Models matter, and testing a million potential chemicals in a test tube doesn't make a dent.
Jack Scannell has an excellent paper going through the decision theory of model quality, one of the core takeaways being "a 0.1 absolute change in correlation coefficient versus clinical outcome can offset large (e.g., 10 fold or greater) changes in brute-force efficiency".

I'm not claiming that developing better models is easy. Research and industry fell into their current SOP because it seemed like a good solution to the problem. There's an alternate universe where what one learns on a cellular assays translates nicely to a mouse which translates nicely to a human. Unfortunately, it's clear that we don't live in that world. The religion of reductionism (I'm looking at you "AI startups for drug discovery") has led to stagnation in higher fidelity models.
Complaining is fun, but what's the solution? We at Transistor Bio are taking a stab at just this problem: drive down the cost of mouse studies. The economic efficiency of mouse studies has not really changed in the last 100 years, but their utility remains the same. By using more complex models with higher predictive power, finding good drugs becomes more tractable. Lowering the barriers to run these studies accelerates the development loop, eventually resulting in a better characterization of the models themselves.
And once you understand what a model says about the biology of humans, well that's just the start.
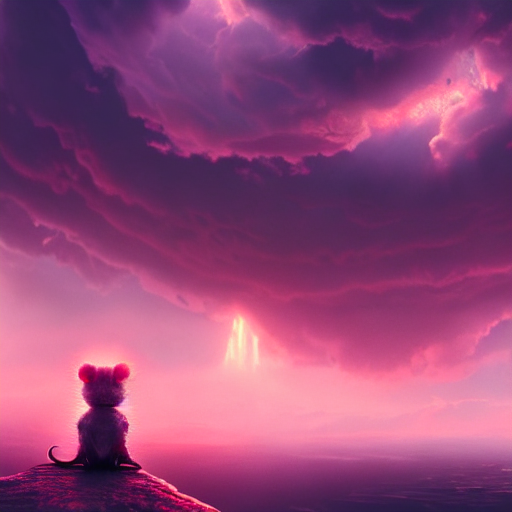